Learning Generalizable Manipulation Policy with Adapter-Based Parameter Fine-Tuning
Kai Lu1, Kim Tien Ly2, William Hebberd2, Kaichen Zhou1, Ioannis Havoutis2, Andrew Markham1
1 K. Lu, K. Zhou, and A. Markham are with the Department
of Computer Science, University of Oxford, Oxford, UK.
2 K. T. Ly, W. Hebberd, and I. Havoutis are with the Oxford Robotics Institute, University of Oxford, Oxford, UK.
Paper Link Code & Data (Coming Soon)
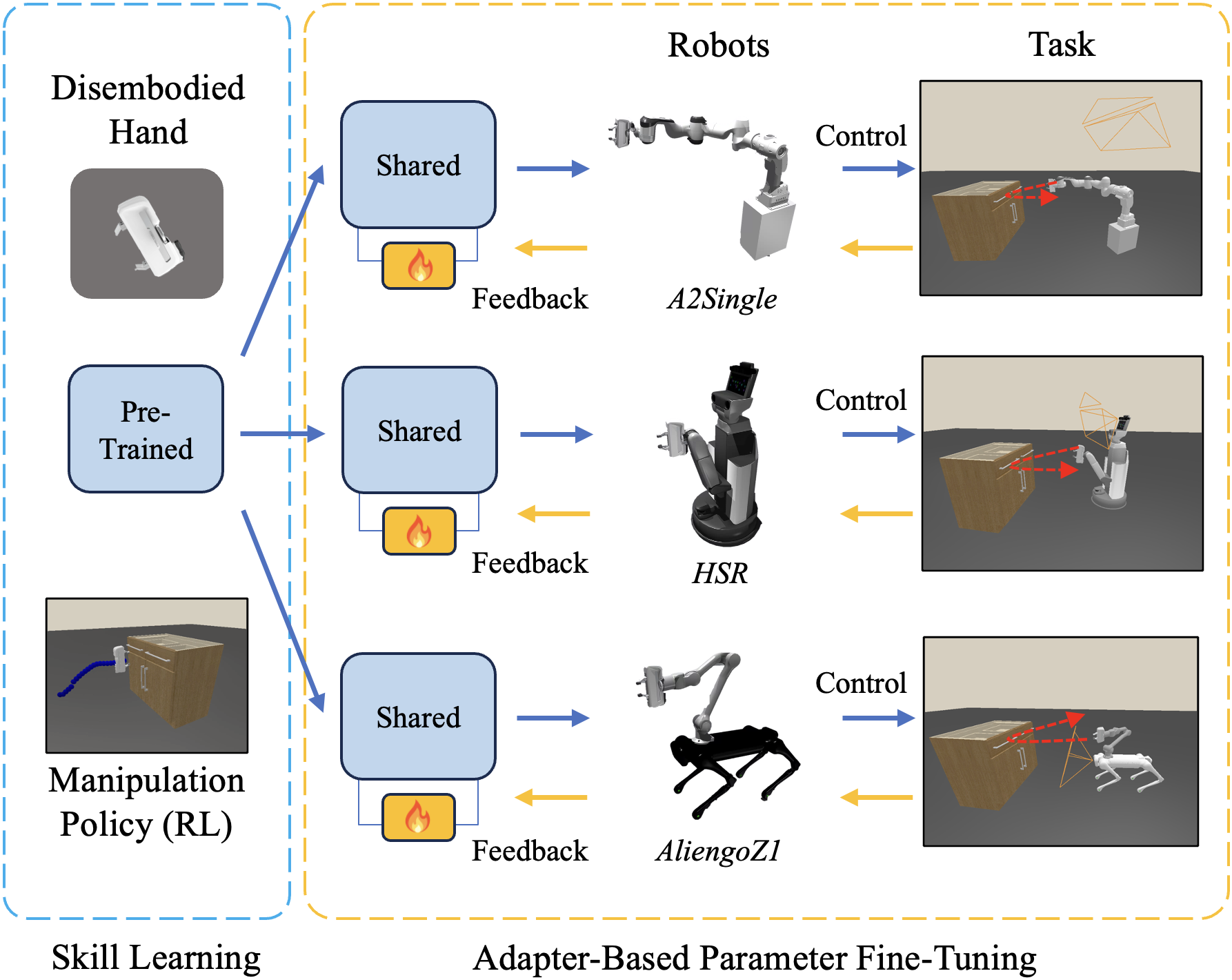
This study investigates the use of adapters in reinforcement learning for robotic skill generalization across multiple robots and tasks. We propose an alternative approach where a disembodied (virtual) hand manipulator learns a task (i.e., an abstract skill) and then transfers it to various robots with different kinematic constraints without retraining the entire model (i.e., the concrete, physical implementation of the skill). Example results in simulation & real (click).
Overview Video
Generalization Across Robots
We present generalizing a learned skill i.e., opening a drawer or a cupboard, to different robotic platforms, including HSR, AliengoZ1, and A2Single.
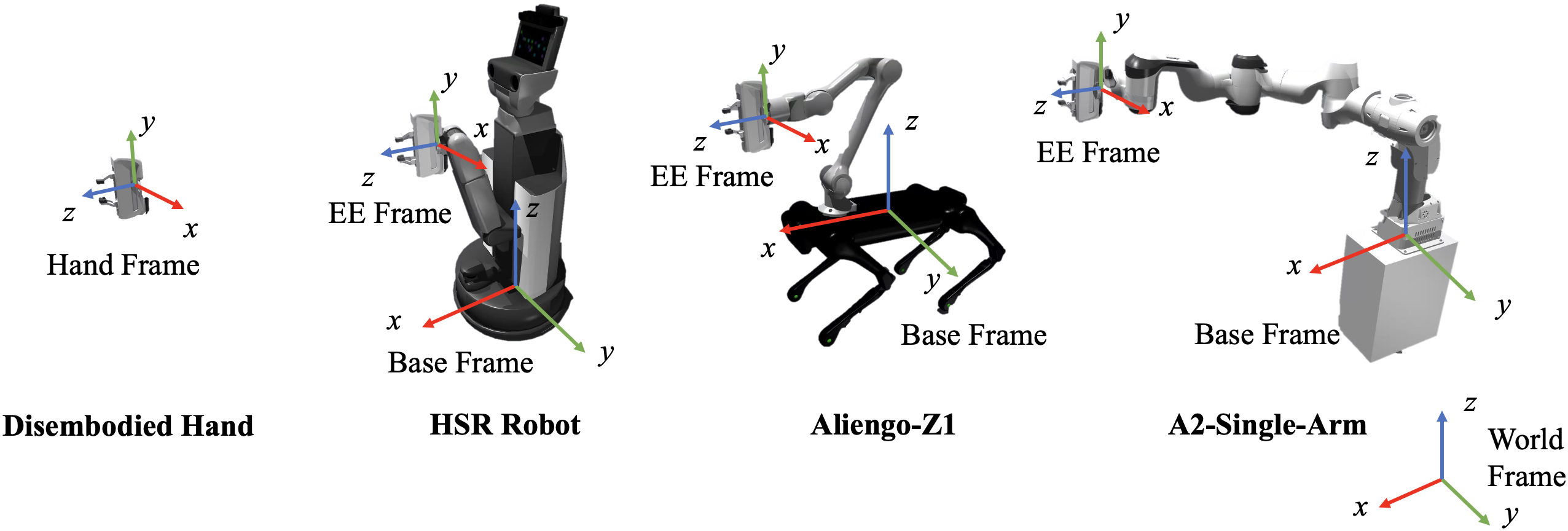
Generalization Across Tasks
We present generalizing a learned skill to different tasks, such as from drawer opening to door opening and chair pushing.
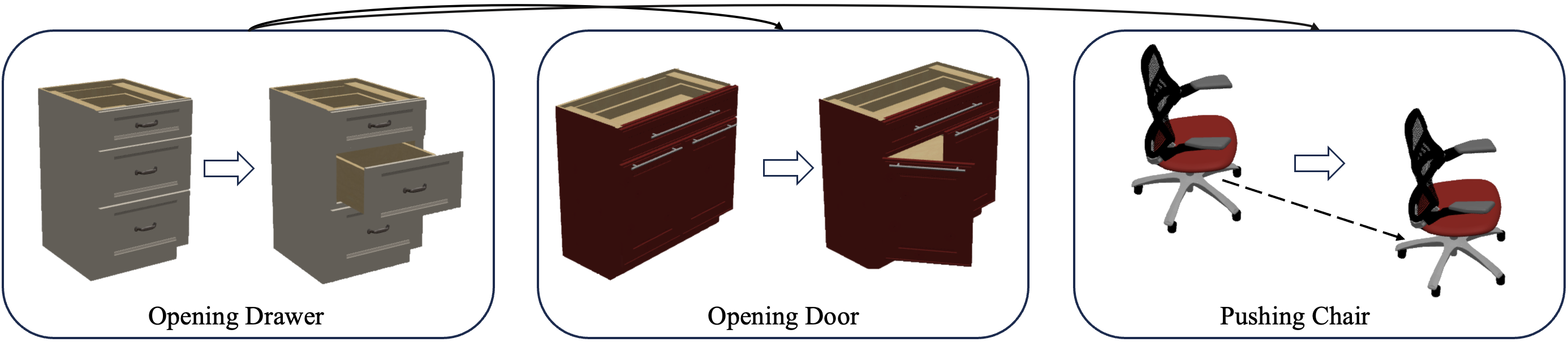
Real Experiments
In the real experiments, we perform the drawer opening task and chair pushing task with a real HSR robot.
The below video shows the chair pushing task.
Contact
Have any questions, please feel free to contact Kai Lu
March, 2024
Copyright © Kai Lu